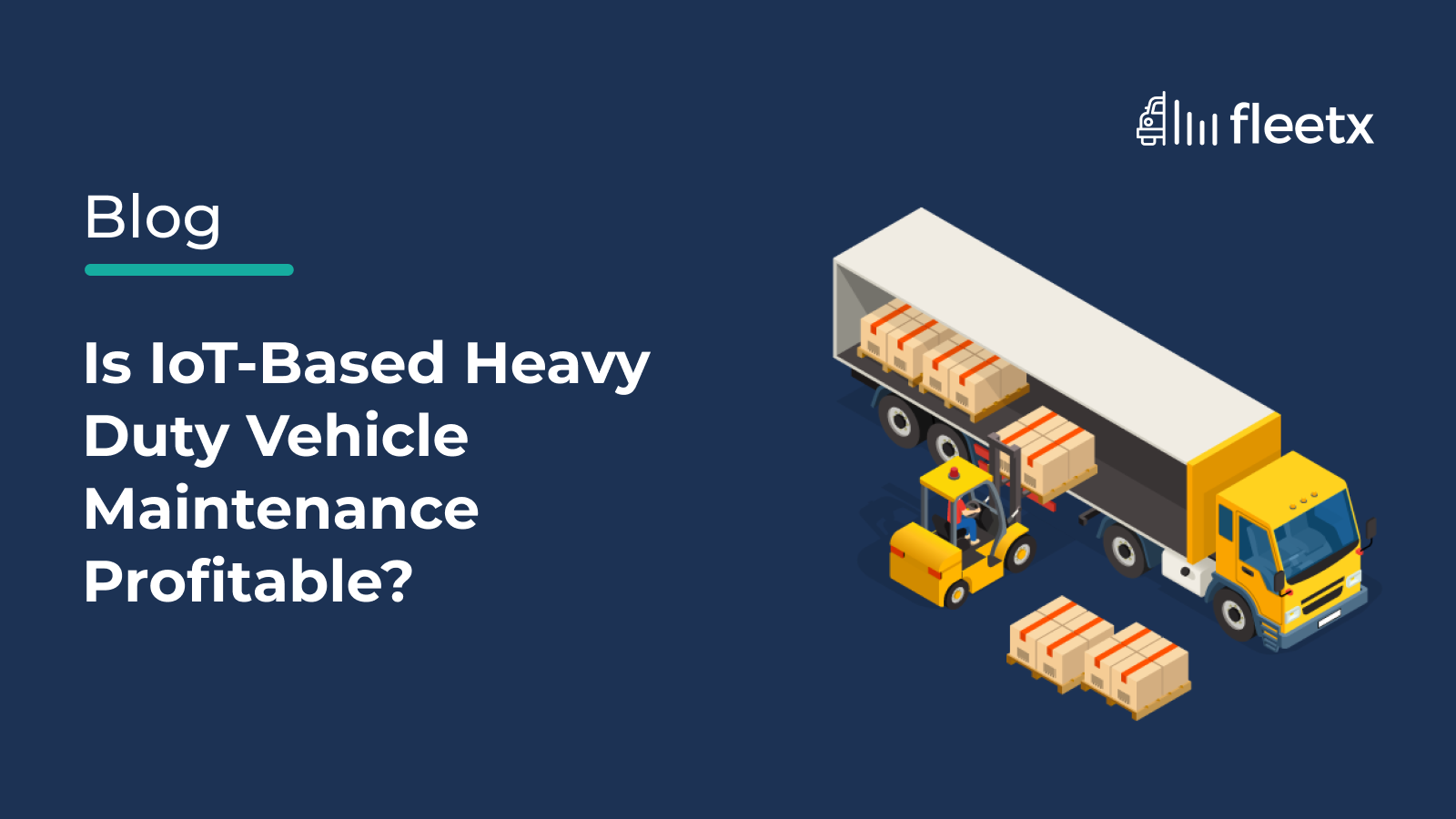
India’s commercial vehicle market is widely segmented, with the primary categories being light, medium, and heavy vehicles. Light commercial vehicles are predominantly utilized in short-distance goods transport, while medium and heavy vehicles transport cargo over long distances, are used in construction sites, and are also utilized as public transportation.
The commercial, heavy-duty vehicle industry is crucial in driving the economy by moving goods and people efficiently and was valued in 2024 at USD 51.27 billion and is expected to reach USD 69.06 billion by 2030, at a CAGR of 5.14%. Adequate vehicle maintenance of such fleets is essential to achieve optimum performance and keep the economy running.
What is Preventive & Predictive Maintenance?
Now, heavy-duty fleets or vehicles need maintenance services to keep functioning smoothly. Vehicle maintenance needs are broadly categorized into preventive and predictive or reactive and proactive, the difference being in the approach. The former is based on predefined schedules and the latter utilizes real-time insights to predict the need for maintenance.
Types of Prescriptive Vehicle Maintenance System
The traditional maintenance methods have been replaced with modern equipment in large vehicles, such as sensors and cameras, to access different data categories using predictive maintenance technologies such as -
- IoT & ML: Leverages vehicle’s sensor data and machine learning algorithms for maintenance
- Vehicle Maintenance Workbench: Combines AI and ML to predict failures and schedule preventive fleet maintenance
- Sound-Based Detection: ML models that recognize patterns and derive the causes of abnormal automotive sounds
- Vehicle Health Management: AI and in-vehicle data are used to warn of potential vehicle malfunctions proactively
- Cloud Solutions: Cloud-based predictive maintenance monitors vehicle components and proactively forecasts potential failures to replace components.
How is PdM an Upgrade Over Traditional Approaches?
The traditional approach is reactive vehicle maintenance instead of proactive. Time-based schedules are necessary but, with widening logistics operations, condition monitoring maintenance is needed.
Reactive to Proactive – By utilizing data and analytics, vehicle component failure can be proactively addressed and unplanned downtime can be avoided.
Higher Efficiency – PdM monitors real-time vehicle updates through IoT for predictive maintenance, ensuring that maintenance is being carried out only when required.
Cost Effective – Resource allocation is optimized by reducing unnecessary maintenance for heavy-duty vehicles and avoiding expensive breakdowns.
Actionable Insights – Leveraging predictive maintenance equipment such as IoT sensors, and technologies like AI, and ML, condition-based monitoring (CBM) offers data like anomaly detection, root cause analysis, and performance optimization for a deeper understanding of vehicle health.
Safe & Compliant – To ensure the vehicle and equipment remain within safe operational limits, predictive analytics reduces risks by maintaining compliance with industry standards.
Scalability & Integrations – Cloud-based PdM solutions can be integrated seamlessly with several ERPs, CRMs, CMMSs, and more to enhance the operational scalability and efficiency of the overall business
Key Features of IoT-Based Predictive Maintenance for Large Commercial Vehicles
An IoT and predictive maintenance software provides –
Real-Time Data - The cloud-based, real-time vehicle and equipment data from the sensors, vehicle telematics, and other sources provide updated insights about the vehicle’s health and performance.
Anomaly Detection – Predictive maintenance equipment identifies data patterns and anomalies to detect potential maintenance-related issues or component failures.
Condition-Based Fleet Maintenance – Maintenance needs are identified based on actual component conditions and not predetermined schedules.
Maintenance Alerts & Notifications – Such systems enable proactive action when AI algorithms analyze historical with real-time data to forecast possible malfunctions and prevent sudden failures.
Workflow of Condition Monitoring Maintenance
The steps involved in executing several types of predictive maintenance are –
Step 1: Data Collection
Vehicle health data is collected from multiple sources including telematics systems, IoT devices, historical data, and other fleet management systems to determine the vehicle performance, usage, equipment health, and environmental impact.
Step 2: Data Preprocessing
The collected data has to be polished and organized into a suitable format that’s easier to analyze further.
Step 3: Feature Engineering
The next stage involves extracting relevant features or vehicle parameters that are indicative of the maintenance status, which may include mileage, fuel consumption, driver monitoring attributes, and others.
Step 4: Data Modeling
Machine learning algorithms or statistical models are used to develop predictive models to generate data-driven estimations for future maintenance needs.
Step 5: Model Training
Historical data with proven outcomes are used to train the model developed in the earlier stage. The AI-based model learns from the historical data patterns to correlate and predict accurately. The trained model is then validated using a separate data set to assess the performance.
Step 6: Real-Time Integration
The generated model is then integrated with live feeds from the vehicle fleets to enable the system to receive, process, and issue real-time updates about the vehicle condition, equipment performance, and several usage parameters.
Step 7: Maintenance Alerts
Combining the features of real-time data and trained models, the predictive maintenance system generates alerts notifying the fleet operators or maintenance in-charge about the specific component maintenance needs or attention required.
Step 8: Data-Based Decision-Making & Corrective Actions
The alerts let the fleet operator make informed decisions and take proactive steps for maintenance plans, resource allocation, and schedule tasks based on urgency and resource availability to minimize vehicle downtime.
Hurdles in Implementing AI-Powered Predictive Maintenance Solutions in Heavy-Duty Fleets
There are several challenges associated with prescriptive maintenance implementation:
- High Initial Investment – Although the long-term savings are significantly high, the cost of sensor installations, AI implementation, and training the personnel would be quite high for small to medium organizations
- Inferior Data Quality – Poor quality or incomplete datasets generate incorrect predictions by the analytical predictive models
- Integration Challenges – AI integration with existing systems can raise big challenges, especially if those systems are not AI-ready
- Lack of Skilled Resources – Implementing and maintaining AI-driven systems requires specialized skills in data science, machine learning, and AI, which is a challenge
- Slow Adaptability – Often, there is resistance toward adopting new technology, particularly with AI. This slows down the process and minimizes the effectiveness
How Do IoT and Predictive Maintenance Convert Into Large Vehicle Fleet Profits?
Maintaining vehicle health of commercial fleets becomes simplified by leveraging AI and it relays into business profits through -
Real-Time Monitoring
AI-driven systems enable continuous vehicle monitoring, including a view into the engine health, tire conditions, fluid levels, and others. The data is analyzed to detect any potential issues. For instance – when a heavy-duty truck indicates engine overheating, AI can flag it for immediate attention.
Proactive Scheduling with Predictive Analytics
Proactive maintenance scheduling before a breakdown occurs is crucial for reducing vehicle downtimes. For instance – an AI model might predict that a heavy-duty vehicle fleet would need brake replacements in the next 1000 kilometers and the fleet operator can schedule the activity accordingly.
Dynamic Maintenance
Following a maintenance schedule based on real-time operational needs and the condition of heavy-duty vehicles provides a level of flexibility in the maintenance schedule that can be deferred or brought forward as required. For instance – a vehicle’s functionality can be maximized by optimizing its maintenance schedule and using it instead for a high-priority job.
Predictive or Preventive - Fleetx Aids Your Complete Vehicle Maintenance
The fleet management system (FMS) from Fleetx has successfully addressed the challenges faced by heavy-duty fleet owners with a module that captures all maintenance needs – from routine maintenance to unexpected repairs. The significant features of the Fleetx service for heavy-duty vehicle maintenance are:
Service History
It consists of the maintenance work done on a vehicle. The common and frequent services are oil change, air filter cleaning, and battery replacement. The associated benefits include:
- Data captured in the service history helps to predict which vehicles are prone to issues and remedial actions can be taken accordingly
- Helpful in reselling old vehicles where buyers need the complete service history
- Aids in vehicle warranty conditions, where the failure to present service records leads to the vehicle warranty being canceled
- Work order issuance and part issuance details
Cost Spent Analysis
The cost spent on the vehicles’ maintenance needs to be monitored regularly to:
- Identify the vehicles whose maintenance cost is higher than their earnings
- Take proactive decisions about vehicle efficiency and replacement
Future Potential of AI in Redefining Commercial Vehicle Maintenance and Fleet Uptime
The role of AI in heavy-duty vehicle maintenance is set to expand. Certain trends that are expected to gain popularity are:
- Autonomous Repair Systems - Self-repairing equipment in the vehicle would be activated by AI to detect and repair minor damages, reducing the need for human intervention
- Adaptive Components - Several component performances can be modified to enhance their usability and condition monitoring maintenance by using AI
- Digital Twins - Virtual replicas of vehicles to simulate wear and tear and failure prediction for real-time optimization suggestions
- Swarm Intelligence - AI-driven fleet management for vehicles to communicate and coordinate repairs for lower fleet downtime
Improved fleet uptime not only boosts productivity but also reduces unnecessary delays and costs. By leveraging the power of IoT, predictive maintenance strategies can turn the insights into actions and create an advanced fleet management system where fleet uptime and efficiency are maximized.